Claassen group, computational systems biology
Infer structure and parameters of biochemical networks through machine learning
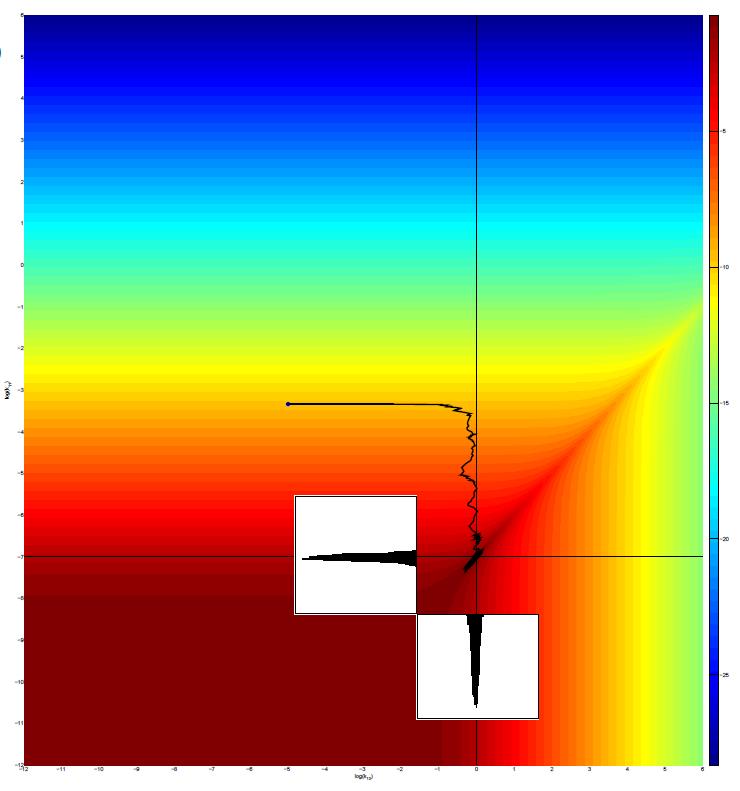
Advances in measurement techniques (like Mass cytometry or sequencing) allow for the simultaneous quantification of multiple biochemical species in high-throughput, single-cell experiments. We develop and apply machine learning methods to infer the structure and parameters of biochemical networks such as those defined by apoptosis and growth factor signaling by utilizing the rich information in such single-cell datasets. For parameter inference, our approach is based on Markov Chain Monte Carlo sampling [1], moment expansion of the Chemical Master Equation [2] and Gaussian Processes [3].
Depending on the background of the applicant, different either more applied or theoretical topics for semester projects or lab rotations are possible, ranging from the application of our method to specific biological systems, evaluating the effects of the additional information in single-cell data or benchmarking our software in comparison to other state-of-the-art methods. All projects will use Matlab. These research projects address students that are new to computational biology and are interested to explore this field, as well as students that aim at deepening their computational biology expertise.
References
[1] Girolami, M. & Calderhead, B. Riemann manifold Langevin and Hamiltonian Monte Carlo methods. J. R. Stat. Soc. Ser. B (Statistical Methodology) 73, 123–214 (2011).
[2] Ale, A., Kirk, P. & Stumpf, M. P. H. A general moment expansion method for stochastic kinetic models. J. Chem. Phys. 138, 174101 (2013).
[3] Rasmussen, C. E. & Williams, C. K. I. Gaussian processes for machine learning. (MIT Press, 2006).